Classe Sra Slot
2016 SRA INDEPENDENT ROWING CARD GUIDE Updated 12-2-2015 Page 3 of 6 AED: SRA is part of the Seattle‐King County Community Responder CPR‐AED Program. Our coaches are SRA first responders but should it be necessary to use the AED without a first responder present, call 9‐1‐1 for instructions. WA has a “Good Samaritan Law” that protects. See Problem with GeneSetCollection after updating R devel packages. And you likely need to update.
THE REEL DEAL
by Frank Legato
Class II: Is It Fair?
Electronic bingo games are becoming more sophisticated and more like traditional slot games.
When the Indian Gaming Regulatory Act of 1988 (IGRA) established the various classes of gaming permissible by Native American tribes on reservation lands, the law designated bingo and similar games under the heading of “Class II.” The classification was established to allow tribes to hold bingo games on their reservations, but it stipulated that “electronic aids” could be used to simulate bingo.
That stipulation led to electronic versions of the game of bingo, which eventually took the form of Class II-style slot machines, known as Bingo Games. The Class II Bingo Games would essentially be a game of bingo, with prizes drawn from the overall money wagered. Those prizes, however, would be displayed as reel results on the slot machines.
In recent years, those Class II bingo machines have become more and more like their traditional, or “Class III,” slot cousins in Las Vegas. The Seminole Hard Rock properties in Florida represent the state of the art in Class II sophistication. The central computer system, developed by a team headed by former IGT systems chief Lyle Bell (now the CIO for the Seminole Hard Rocks), was created with a singular purpose—to provide a player’s-club experience to simulate the Class III slot experience on a Class II floor. Meanwhile, Casino Operations Senior VP, Charles Lombardo—formerly slot operations VP at Caesars Palace—worked with the major slot manufacturers, who refined Class II technology to provide games that look and play like the traditional games.
Though they are technically electronic bingo games, the Hard Rock’s slots mimic the traditional Las Vegas-style games in every way. Other than the LCD screen that shows the bingo patterns appearing with every spin, it is hard to tell the difference.
How do they make bingo games behave like slot machines? And how are the payback percentages determined? The answer to both can be summed up in one word: mathematics. The Class II electronic bingo games at the Hard Rock are programmed with mathematical calculations to mimic Class III games as closely as possible while remaining within the definition of Class II bingo that is contained in IGRA.
Under IGRA, a Class II game must have a draw of bingo balls, and must result in what is called a “game-ending pattern.” That is a pattern of numbers—two, three, four in a row; diagonal, vertical, four corners of the bingo card, etc.—that ends the game with a winning result.
According to Lombardo, this occurs continuously. “We have a 20-millisecond window, and anyone (in the casino) pushing the Play button during that window is put in the game for that common ball draw,” he explains. “It must be at least two players, but the maximum is unlimited. If it is a minimum of two, one of them gets a bingo—a winning pattern.” He says every ball draw results in at least one bingo.
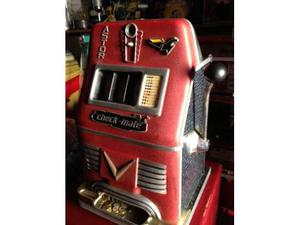
How do the payback percentages work? One of two ways, says Lombardo. In one style of game, the calculations relate to the stack of possible outcomes loaded into the central computer. In this style of game, there is always a 50-percent hit frequency—one of every two spins on average results in a bingo, with a prize determined from a finite pool of outcomes loaded into the computer. When the bingo game is over, the computer selects a prize from the top of an electronic “stack” and feeds it to one of the games with a winning outcome. To the player, it looks, for instance, like a mixed-bar win for $5. That just means a $5 prize has been awarded from the results of the bingo game.
The overall payback percentage in this case is governed by how many results equal to each prize amount are included in the finite stack of prizes. Just like the universe of numbers from which the random number generator in a regular slot selects reel outcomes, the payback percentage here is determined by the universe of prizes available for each winning result. The hit frequency is always 50 percent, but the payback percentage is determined by how many $2 prizes, how many 75-cent prizes, how many $1,000 prizes, and so on, are loaded into the program.
In a multiline video bingo game, this system results in a game virtually indistinguishable from that nine-line game in the Vegas casino that has a 50-percent hit frequency. According to Lombardo, though, this method is also used on some of the traditional single-line, three-reel slots. In this case, the 50-percent frequency still stands, but not every win is a traditional reel combination. Because traditional games like Blazing 7s or Red, White & Blue generally have hit frequencies around 14 percent for the seven or eight possible winning combinations in the pay schedule, a 50-percent frequency would be impossible and still have the game make money for the casino.
To remedy this, Lombardo explains, “we came up with a bonus feature.” Fourteen-percent of results in the pool will be actual reel combinations, and the other 36 percent of the winners will yield a bonus symbol on the reels that will accumulate. When you accumulate 25 of those symbols, you win one bonus credit. Therefore, you still have the 50-percent frequency, but your frequency of reel wins is similar to what it is in the traditional Class III versions of those games.
In the other style of game, the odds of each winning bingo pattern is matched to the odds of each paying combination in the slot game. “We figured out the odds of hitting certain patterns on the bingo card,” Lombardo explains, “and we take those bingo patterns and plug them right into the payout scheme to replicate any Class III game.” Drawing from millions of possible patterns on a bingo card, programmers can match the odds of landing any given combination of symbols on a slot machine. In this way, each chosen bingo pattern can trigger a certain payout combination. Hit frequencies and percentages in this case will match a traditional slot exactly.
But what are those payback percentages, and how do we know they are fair? As you may know, the Seminole tribe is a sovereign nation, and its casinos are not subject to state regulation or public reporting of payback percentages. How do we know we’re getting a fair shake?
We know we’re getting a fair shake because tribal casinos must compete with all other casino choices, says Lombardo. “We are competitive with all Class III markets,” he says. “We’re not doing anything differently (with percentages) than Atlantic City, Las Vegas or Mississippi. We are competitive with any casino in the country.” He adds that he takes average bets in lower denominations into account when determining the payback percentage he wants to offer. “If I am requiring players to cover the lines on a 20-line nickel game, that’s a dollar bet,” says Lombardo. “I take that into consideration when I figure out the payback percentage I offer.”
Lombardo adds that tribal casinos have obligations to both the players and the slot manufacturers to keep the games fair. “Over the long hall, any player is going to know if you screw with percentages; they’ll know the difference,” he says. “And, a manufacturer is not going to give us their title if we are going to misrepresent that title (with low payback). We don’t want to kill a title.”
It is that respect for the player—and obligation to represent a manufacturer’s title fairly—that should make you approach the slot experience at the Hard Rock or other large Class II tribal casinos with expectations similar to those you have when playing slots in most major jurisdictions. In other words, you are likely to get a fair shake. They know that if you don’t, you will go elsewhere.
TIP OF THE MONTH
Class II Video Poker
We have noted before that video poker in a Class II tribal casino does not work in the same manner as video poker in a traditional casino. While this is true, it does not mean that it is unfair, or that you can’t win.
The result of any Class II video poker hand is predetermined by the result of the ball draw in the bingo game on the little screen. In some jurisdictions, you will be required to touch the screen to daub the bingo card and claim your prize.
Those results are determined by winning patterns on the bingo card. Using one of the two methods described above, a winning pattern will either trigger one of a stack of predetermined prizes or a corresponding video poker hand, according to the odds. The odds are calculated to be similar to the odds of a standard video poker game. You won’t find the player’s-advantage paytables in Class II, but the return represented by the pay schedule you do see will be similar to the return of that game in a traditional casino.
The real difference lies in the importance of perfect strategy. A Class II video poker game is actually better for the strategy novice, because the game will often correct your bonehead moves. If the bingo result determines you win the a certain prize, you will get that prize even if you make the wrong choice, through a special feature on the game.
For instance, on the IGT version of Class II video poker, let’s say your winning bingo pattern translates to the prize for four-of-a-kind, and you are dealt 10-c J-c Q-c J-h J-s. Even if you screw up and decide to go for the royal, the game will not let you. A “Genie” will appear on the screen and change your hand to four Jacks—for the quad prize that corresponds to the bingo pattern you got.
It’s better for the novice because it shows you the optimal strategy by changing your choice to match the bingo win. Other than that, the Hard Rock’s Lombardo says the games work like the standard versions of video poker—again, because the Class II casino will not risk “killing a manufacturer’s title.” “A lot of players may not know the difference between Class II and Class III,” he says. “That’s why we replicate the video poker paytables as closely as possible. It would be a killer for us to do anything else.”
2019-10-01

- 2 Stock Reduction Analysis (SRA) for conditioning DLMtool operating models
- 4 Mathematical description of the SRA model
For conditioning an operating model, it is desirable that some objective method be available (as opposed to intuition or a simple guess) to inform key historical parameters. Biological studies can be used to inform life history parameters such as growth and maturity, while other parameters such as depletion and fishing mortality have typically been informed by an assessment model. In) or effort. If the model is conditioned on catch, then the SRA will generate predicted catches that match the observed. If conditioned on effort, the estimated fishing mortality in the model will be proportional to the observed effort. A full time series of the conditioning variable is needed, and length of the historical period OM@nyears
will be the length of the conditioned time series.
Classe Sra Sloth
Ideally, the time series begins at unfished conditions. One could pass the asssumed equilibrium catch or equilibrium effort prior to the first year of data to SRA_scope
. The SRA will then attempt to estimate the initial depletion in the first year of the historical period. However, this is generally difficult to estimate in the first place (consider what data are informative to estimate initial depletion, perhaps an age or length sample from that first year that shows the truncation of the composition data relative to unfished conditions).
If catch or effort data are unavailable going back to unfished conditions, then the data could be extrapolated back in time using reconstruction. Examples of catch reconstruction methods for the purposes of a stock asesssment can be found in Porch et al. (2004) and Appendix A of Starr and Haigh (2017).
In addition to the conditioning variable, additional data types can be used: - Indices of abundance (either as surveyed biomass or fishery-dependent catch-per-unit time series) - Age compositions - Length compositions - Mean lengths (this option is generally for very sparse data scenarios when mean length data are available but not the composition data)
Multiple surveys and fleets can be accommodated with SRA_scope
. One of these several data types in addition to catch or effort is generally needed to obtain depletion estimates. Availability of these data can be quite sparse over time, yet still informative. For example, a recent age composition sample from a single year that shows a very truncated age structure can sufficient to imply a heavily depleted stock.
Classe Sra Slot Car
Here are the required pre-specified OM parameters needed for SRA scoping:
- Growth (length-at-age) using slots
OM@Linf, OM@K, OM@t0
(or alternatively,OM@cpars$Len_age
) andOM@LenCV
- Length-weight conversion factors using slots
OM@a
andOM@b
- Natural mortality using slots
OM@M, OM@M2
orOM@cpars$M_ageArray
- Maturity using slots
OM@L50, OM@L50_95
orOM@cpars$Mat_age
- Standard deviation of recruitment deviations using slot
OM@Perr
orOM@cpars$Perr
- Stock-recruit relationship with
OM@SRrel
- If no age or length compositions, selectivity is needed with
OM@L5, OM@LFS, and OM@Vmaxlen
If growth, natural mortality, or maturity are time-varying in the historical period, then the SRA will implement time-varying life history in the estimation model as well. For example, we’re setting up an operating model where the length of the historical period is 50 years, and we believe that natural mortality has doubled from 0.2 to 0.4 since Year 30 and will remain so into the future. This code can be used to setup this scenario:
The SRA will pick up this change in the model as well.
Note that time-varying life history affects calculation of reference points, in particular unfished depletion. The SRA_scope
function will annually calculate the unfished depletion associated with that year’s life history values. The easiest way to turn off time-varying growth and M is to set:
Selectivity is fixed if no age or length compositions are provided. Otherwise, the ascending limb of selectivity is estimated with age or length composition data. If the selectivity is assumed to be dome-shaped, then the descending limb can either be fixed values sampled from slot OM@Vmaxlen
or estimated in the SRA.
Information about the slots in the OM object can be viewed through class?OM
. If passing custom objects to the operating model that override default inputs (e.g., for time-varying parameters), then DLMtool::validcpars()
will be helpful for setting up and indexing the dimensions of the custom objects.
2.2 Updated parameters and SRA model evaluation
Historical OM parameters that are updated by the SRA scoping function include:
- Unfished recruitment
OM@R0
, only if catch is provided - Initial depletion
OM@cpars$initD
- Depletion
OM@D
- Relative effort
OM@cpars$Find
. - Annual recruitment deviations
OM@cpars$Perr_y
. - If age or length compositions were used, selectivity parameters
OM@L5, OM@LFS, and OM@Vmaxlen
.
The SRA model will estimate and return R0
when conditioned on catch. When conditioning on effort, the model is generally scale-independent; there can be information to inform depletion but not the stock size.
The exception occurs when the SRA is conditioned on effort from multiple-fleets, in which case, catch data from all fleets are needed in inform the relative F’s among fleets. In this scenario, R0 is estimated.
Additionally, if multiple fleets are used for conditioning, then the annual selectivity can change basd on the relative F among fleets. In this case, the annual selectivity is passed the OM output in the OM@cpars$V
slot. The default assumption in the projection period of the closed-loop simulation is that the selectivity and relative F among fleets are identical to those in the last historical year. Fleet allocation in management procedures can be exploration in multiMSE
, see vignette('multiMSE')
.
The relative effort provided in the output is the apical F from the SRA. When running the management strategy evaluation with DLMtool::runMSE()
, the apical F may be re-scaled to ensure that specified depletion has been reached at the beginning and end of the historical period. For simple operating models, i.e. those with conditions identical to the SRA, the apical F’s in the MSE should be nearly identical to those from the SRA. To confirm that this is the case, one can run the plot
function on output returned by SRA_scope
:
This function returns a markdown report with:
- Histograms of updated parameters in the OM object
- Fits of the SRA model to the provided data
- Output from the SRA model, e.g. predicted recruitment
- Fits to an additional run of the SRA model to mean life history values among simulations (only when
SRA_scope(..., mean_fit = TRUE)
is run) - Comparisons of the historical period of the updated OM to the SRA output
Currently, it is possible to create a more complex operating model than the SRA model itself. For example, discard mortality, movement, and spatial targetting are currently not modeled in the SRA. If these processes are specified in the operating model, then it is possible that the operating model may not necessarily match all output from the SRA.
To be added later.
4.1 Dynamics equations
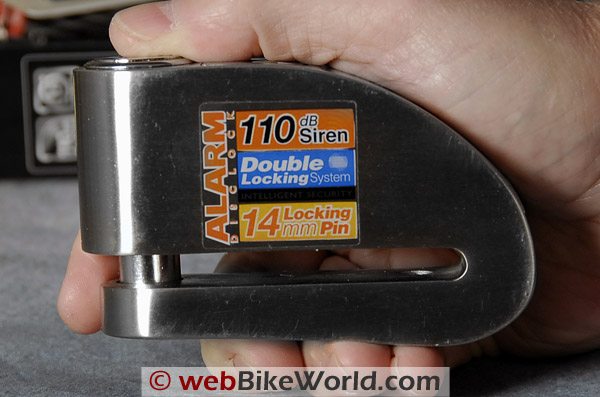
Selectivity (v) for length bin (ell) and fleet (f) is length-based, where [ v_{ell,f} = left[1 + expleft(-log(19) left(dfrac{L^{50}_f - L_{ell}}{L^{95}_f - L^{50}_f}right)right)right]^{-1} ] if logistic shaped, with (L) is the midpoint of length bin (ell) and (L^{95}_f) and (L^{50}_f) are the lengths at 95 and 50% selectivity, respectively.
If dome shaped, selectivity is [ v_{ell,f} = begin{cases}expleft(-dfrac{(L_{ell} - mu_f)^2}{2(sigma^L_f)^2}right) & L_{ell} < mu_fexpleft(-dfrac{(L_{ell} - mu_f)^2}{2(sigma^R_f)^2}right) & L_{ell} ge mu_fend{cases},] where (mu_f) is the length of full selectivity and (sigma^L_f) and (sigma^R_f) control the shape of the ascending and descending limbs, respectively, of the selectivity function.
Total mortality (Z) in year (y) and for age (a) and length bin is the sum of fishing mortality (F) from all fleets for that length bin and natural mortality (M) for that age,
[ Z_{y,a,ell} = M_{y,a} + Sigma_f v_{ell,f} F_{y,f}.] The catch (in numbers) (C^N) at age and length for fleet (f) is [ C^N_{y,a,ell,f} = dfrac{v_{ell,f} F_{y,f}}{Z_{y,a,ell}} N_{y,a,ell} (1 - exp[-Z_{y,a,ell}]).]
If the model is conditioned on catch, then (F_{y,f}) are estimated. If the model is conditioned on effort, then [ F_{y,f} = q_f E_{y,f},] where (E_{y,f}) is the observed effort and (q^f) is a scaling coefficient.
The population abundance (N) at age and length is calculated assuming a normally distributed length-at-age (P(ell,a)), where [ N_{y,a,ell} = N_{y,a} P(ell a) ] and
[ P(ell a) = begin{cases} phi(L'_{ell+1}) & ell = 1 phi(L'_{ell+1}) - phi(L'_ell) & ell = 2, ldots, L - 1, 1 -phi(L'_ell) & ell = Lend{cases}] with (L'_{ell}) as the length at the lower boundary of length bin (ell) and (phi(L'_{ell})) as the cumulative distribution function of a normal variable with mean (tilde{L}_{y,a}) (the expected mean length at age (a)) and standard deviation (tilde{L}_{y,a} times CV^L) ((CV^L) is the coefficient of variation in mean length at age).
After setting the equilibrium population age distribution in the first year of the model, the population abundance (after summing across all length bins) in subsequent years is [ N_{y,a} =begin{cases} R_y & a = 1 Sigma_{ell} N_{y-1,a-1,ell} exp(-Z_{y-1,a-1,ell}) & a = 2, ldots, A - 1, Sigma_{ell} N_{y-1,a-1,ell} exp(-Z_{y-1,a-1,ell}) + Sigma_{ell} N_{y-1,a,ell} exp(-Z_{y-1,a,ell}) & a = Aend{cases}] where (R_y) is the recruitment predicted in year (y).
The catch in weight (tilde{C}) is [ tilde{C}_{y,f} = Sigma_{ell}Sigma_a C^N_{y,a,ell,f} w_{ell},] where (w) is the weight at length bin (ell).
Classe Sra Slot Cars
The mean length of the catch (bar{L}_{y,f}) is [ bar{L}_{y,f} = dfrac{Sigma_{ell} L_{ell}Sigma_a C^N_{y,a,ell,f}}{Sigma_aSigma_{ell} C^N_{y,a,ell,f}}. ]
The proportion of the catch-at-age is [ p_{y,a,f} = dfrac{Sigma_{ell}C^N_{y,a,ell,f}}{Sigma_a Sigma_{ell}C^N_{y,a,ell,f}}.]
The proportion of the catch-at-length is [ p_{y,ell,f} = dfrac{Sigma_a C^N_{y,a,ell,f}}{Sigma_a Sigma_{ell}C^N_{y,a,ell,f}}.]
The spawning biomass is (B^S_y) is [B^S_y = Sigma_{ell} w_{ell} Sigma_a m_a N_{y,a,ell},]
where (m_a) is the maturity at age.
4.2 Likelihoods
If the model is conditioned on catch, then the log-likelihood component (Lambda_1) of the catch is [Lambda_1 = Sigma_y Sigma_f left(-log(0.01) - dfrac{[log(tilde{C}^{textrm{obs}}_{y,f}) - log(tilde{C}^{textrm{pred}}_{y,f})]^2}{2 times 0.01^2}right),]
where (textrm{obs}) and (textrm{pred}) indicate observed and predicted quantities, respectively. With a small standard deviation for the catch likelihood relative to the variance in other likelihood components, the predicted catch will generally match the observed catch.
The log-likelihood component (Lambda_2) of survey data is [Lambda_2 = Sigma_y Sigma_s left(-log(sigma_{y,s}) - dfrac{[log(I_{y,s}) - log(q_s B_{y,s})]^2}{2sigma_{y,s}^2}right),] where (I) is the value of the survey with (s) indexing survey, (sigma_{y,s}) is the corresponding standard deviation of the survey, (q) is a scaling coefficient, and (B_{y,s}) is the reference biomass that corresponds to the index, e.g., spawning biomass or vulnerable biomass.
The log-likelihood component (Lambda_3) of catch-at-age data is [Lambda_3 = Sigma_y Sigma_f O^A_{y,f} log(p_{y,a,f})] where (O^A) is the annual sample sizes for the age compositions.
The log-likelihood component (Lambda_4) of catch-at-length data is [Lambda_4 = Sigma_y Sigma_f O^L_{y,f} log(p_{y,ell,f})] where (O^L) is the annual sample sizes for the length compositions.
The log-likelihood component (Lambda_5) of observed mean lengths in the catch is [Lambda_5 = Sigma_y Sigma_f left(-log(omega_f) - dfrac{[tilde{L}^{textrm{obs}}_{y,f} - tilde{L}^{textrm{pred}}_{y,f}]^2}{2 omega^2_f}right),] where (omega_f) is the standard deviation of mean lengths.
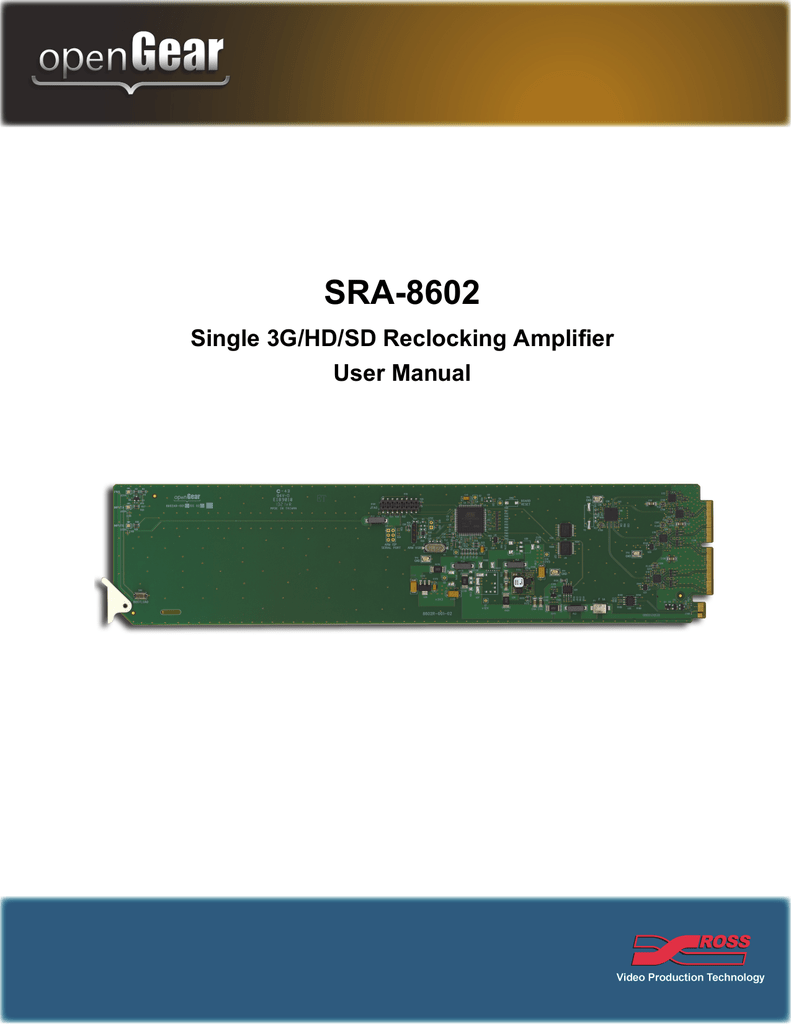
The log-likelihood component (Lambda_6) of annual estimated recruitment deviates (delta_y) in log space is [Lambda_6 = Sigma_yleft(-log(tau) - dfrac{delta_y^2}{2 tau^2}right),] where (tau) is the standard deviation of recruitment deviates.
Kimura, D.K. and Tagart, J.V. 1982. Stock Reduction Analysis, Another Solution to the Catch Equations. Can. J. Fish. Aquat. Sci. 39: 1467-1472.
Porch, C.E., Turner, S.C., and Schirripa, M.J. 2004. The commercial landings of red snapper in the Gulf of Mexico from 1872 to 1962. SEDAR7-AW-22. SEDAR, North Charleston, South Carolina. Available at: http://sedarweb.org/docs/wpapers/SEDAR7-AW-22.pdf (Retrieved July 9, 2019)

Starr, P.J. and Haigh, R. 2017. Stock assessment of the coastwide population of Shortspine Thornyhead (Sebastolobus alascanus) in 2015 off the British Columbia coast. DFO Can. Sci. Advis. Sec. Res. Doc. 2017/015. ix + 174 p. Available at: http://www.dfo-mpo.gc.ca/csas-sccs/Publications/ResDocs-DocRech/2017/2017_015-eng.html (Retrieved July 9, 2019)
Walters, C.J., Martell, S.J.D., and Korman, J. 2004. A stochastic approach to stock reduction analysis. Can. J. Fish. Aquat. Sci. 63: 212-223.